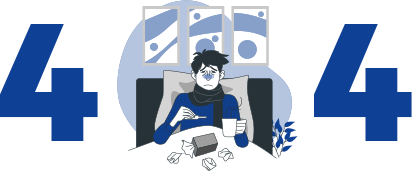
Oops! Page Not Found
The page you are looking for might have been removed, had its name changed, or is temporarily unavailable.
Go to HomepageThe page you are looking for might have been removed, had its name changed, or is temporarily unavailable.
Go to Homepage